NEGOTIATING THE PANDEMIC TWITTERVERSE
Muhammad Abdul-Mageed | March 8, 2021
Muhammad Abdul-Mageed is Assistant Professor in both the School of Information and the Department of Linguistics at the University of British Columbia
Society’s increasing reliance on social networks since the start of pandemic raises important questions about the role of these networks in our pandemic lives. To investigate some of these questions, we have been studying publicly available messaging data on Twitter.
When we started this research, we had a number of questions: Has the pandemic changed any general patterns of communication online? What major functions is Twitter playing during the pandemic? Can the data be used to measure our restricted physical activity? How much false information is shared on the network?
To understand the impact across language and culture, we collected a diverse 10-year dataset from one and a half billion public Twitter messages posted by users from 286 countries in 100+ languages so that we can compare before and during COVID-19. The messages exchanged between users are themselves an important archive of life during the pandemic that can reveal new knowledge about human behavior, including how individuals and groups are coping around the globe. Thus, extracting opinions and summarizing trends from this public data, while respecting privacy, can help guide policy makers to better understand what the public needs and what the best ways are to serve different communities.
The pandemic sharply changed the usual flow of communication on Twitter. For example, during the first quarter of 2020 the most frequent activity was direct interactions between users rather than the typical posting of tweets. Compared with older data between 2007-2019, it is clear that the pattern of users engaging in conversations is associated with the pandemic. For the first time in the history of Twitter, users are more interested in directly talking to one another than in sending tweets.
We found that users not only reached out to others to offer sympathy, talk about family, and relate to life events but that conversations also included heated discussions about government policies, workplace accommodations, access to services, and other important topics.
We derived other insights from this data. For example, during the first three months of 2020, in Europe where some countries were being hit quite hard, pandemic related messages were more frequent while in Asia more conversations involved political discussions unrelated to the pandemic. Such information about what people in a particular region care about at a given point in time, and their identifiable level of awareness about the health crisis, could be used to allocate resources and carry out targeted information campaigns. Artificial intelligence (AI) can power technologies of this type of opinion mining, enhancing, or even replacing, traditional polling methods such as questionnaires and phone call surveys.
The data also allowed us to gain insight into human physical activity as many users chose to share their location publicly and/or talked about places they visited. We found that many people reduced their activity levels starting in March 2020 but globally the activity patterns followed the pandemic. For example, the decline in activity started in Italy earlier than in the U.S. and Canada indicating how social data match human activity on the ground. Since these patterns can be acquired while events are happening, they can be used to help guide timely public health policy.
We also used the data to identify and quantify COVID misinformation on the network using a two-stage approach. We first taught computers to detect whether a tweet was about the pandemic or not, and any new incoming post related to COVID was examined by another AI model to determine whether the post is “true” or “false”. True posts are simply those that do not contradict known facts, while false ones are those that carry rumors and fake stories about the pandemic. The model can spot false pieces of text such as “Corona virus can be cured by one bowl of freshly boiled garlic water…. doctor has proven its efficacy” and “Drinking alcohol is the best remedy for COVID”. Using this detection approach, we quantified misinformation on the network using 30 million tweets randomly sampled from data not used to develop the model and found that about 2.5% of all English tweets posted in early 2020 carried misinformation about the pandemic. While this might seem small, we estimated that over 7 million tweets with COVID misinformation were shared every single day in the first half of 2020. Even if each tweet is seen by only 150 people on the network, this amounts to over one billion reads. The World Health Organization was correct to label this situation an infodemic due to the rapid spread of false information. It only takes a single false tweet acted upon by only a few people for someone, or many people, to be hurt. As a society, we need to proactively work to address the infodemic. But how?
The first thing we need to do is equip people with the critical thinking and research skills to identify, question, and evaluate what they see online.
We also need to encourage Canadians to actively refute misinformation when they witness it, civilly and without alienating others. For example, a message such as “Thank you for your post. I found this page from WHO that emphasizes garlic cannot cure COVID. Grateful to connect here!” is informative while being friendly. Individuals need to be able to locate evidence and make informed judgements as they navigate through their daily information journey.
In order to employ resources at a more societal level, investments in deep learning within the research community as well as by provincial and federal governments could better equip Canadians to combat the Infodemic.
This article initially appeared in the Globe and Mail on March 5, 2021.
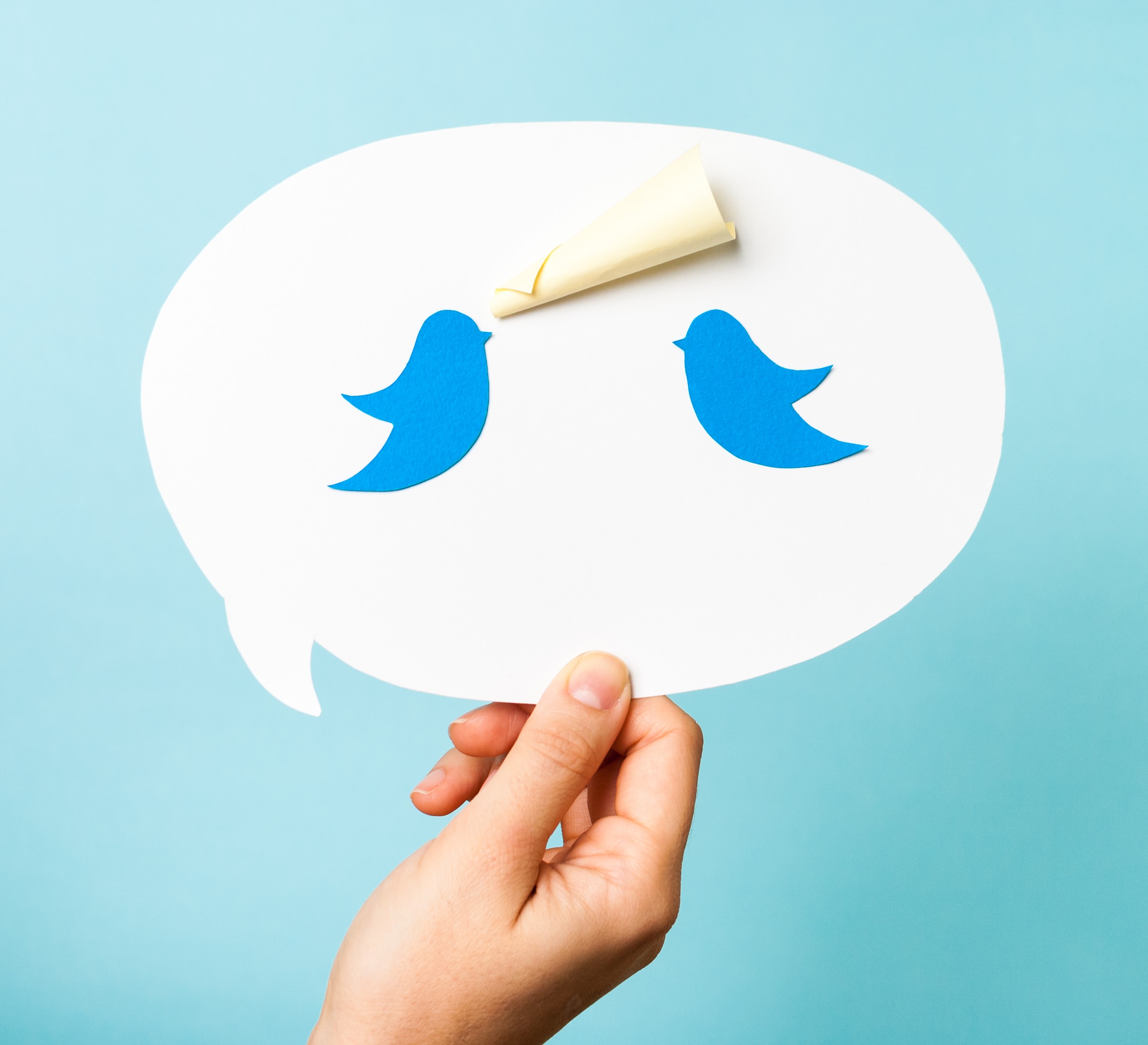