
MODELS IN THE TIME OF COVID-19
Mark A. Lewis and Daniel Coombs | May 28, 2020
The global COVID-19 pandemic that has unfolded around the world over the last six months has drawn attention towards quantitative mathematical modelling as never before. Government policymakers and the general public alike are looking towards science, and modelling in particular, to understand the complex dynamics of the epidemic from local to global perspectives, as well as to project the impact of possible interventions on numbers of cases, hospitalizations and deaths. Modelling has played a crucial role, as we have witnessed in daily updates from our chief medical officers, government officials and elected leaders.
A particularly noteworthy example is the model used by Neil Ferguson and colleagues from Imperial College, London, to advise the UK government of the likely impact of allowing the disease to spread unchecked [1]. The changes in policy towards social distancing, influenced by these modelling results, almost certainly saved thousands of lives in the UK and supported the adoption of similar policies in the USA.
The information flowing from any model is limited, and many (indeed most) modelling groups are quick to point out the shortcomings of their own work and those of others. The highly influential Imperial College model relied on estimates of parameters that were, and still are deeply uncertain.
Should we be concerned? That depends on what we care about. If we care about devising short-term strategies to combat the most devastating pandemic in recent history, then the short answer is “no.” The Imperial College model, and others like it, have amply demonstrated the need for social distancing and the catastrophic consequences of not doing so, a beautiful illustration of the often-repeated mantra: “All models are wrong, but some models are useful.” However, if we care about making more accurate long-term predictions so we can plan how to restart the economy, the answer is “we can do better.” We can do better because we now have more data, which allows us to develop new kinds of models.
Mathematical models for infectious disease dynamics have been around for a long time. Mathematician Daniel Bernoulli developed a dynamical model for smallpox transmission and control in 1760 [2]. Ronald Ross, a medical doctor and Nobel Prize winner with mathematical inclinations, developed a mathematical theory for the outbreak dynamics of malaria in 1911 [3].
Two decades later, W.O. Kermack and A.G. McKendrick collaborated to develop a new theory of infectious disease transmission [4]. Their model broke up the population into susceptible (S), infected (I) and recovered/removed (R) groups. This allowed them to focus on the rates of transfer of people between groups based on the biological course of infection, transforming S-individuals into I-individuals and eventually to the R group.
The SIR model employed a very simple idea: that of infection by “mass action,” which is akin to assuming that each random encounter between susceptible individuals and infected individuals has a certain chance of resulting in new infection. They used their model to achieve a new understanding of the 1906 Bombay plague epidemic.
This style of “compartmental modelling” has stuck with us, and the basics are taught to epidemiologists and applied mathematicians in undergraduate classes around the world. Although the approach is simple, it has been remarkably successful in explaining patterns of infection outbreak, including outbreaks of the flu in British boarding schools. Extensions include more classes of individuals (e.g., Exposed, Quarantined or Asymptomatic), as well as differential risks for different groups (e.g., those with differential risk of contracting AIDS based on sexual encounters). Indeed, the structure of many modern epidemic models for COVID-19 are based on modifications of the SEIR compartmental structure, with the additional Exposed class inserted between Susceptible and Infected.
These models give rise to a key concept in the study of epidemics, the basic reproduction number (R0), which is the average number of new infections coming from a single infected individual. R0 larger than one means the disease can grow exponentially, with possibly devastating consequences. However, R0 smaller than one means the disease should die out. Thus, reducing R0 becomes an essential management goal. For the Kermack-McKendrick model, R0 is the rate at which a single infected individual infects others, multiplied by the period of infectiousness. Social distancing can reduce the first, while quarantining shortens the second. Both control strategies therefore become powerful methods to reduce R0 and hence to drive down disease levels. However, the value of R0 can change daily in step with changes in social policies. Thus, when daily case counts are available, we can compute the daily values of R0 that make the model outputs closest to the measured data. This process, called model fitting, is how most published estimates of R0 for the COVID-19 pandemic have been produced. By simultaneously fitting essential parameters to available data, the model gradually becomes a tool for projecting the future course of the epidemic. In the current context, we can use this approach to make projections of hospitalization or intensive care admission rates during the epidemic, and to advise officials to prepare accordingly.
One would be tempted to think that the more realistic a model is, the better it is. So, if we want better dynamical models, should we just make them more complicated? Here, a classic dilemma of modelling arises. The more realistic, and therefore complex, a model is, the more uncertain the outcome. This is because the behaviour of a complex model depends upon a myriad of detailed input parameters, many of which are unknown or only known approximately. Indeed, there is always a trade-off between accuracy (how realistically the model incorporates all the different possible inputs) and precision (the level of certainty associated with the model predictions). The problem with COVID-19 is that some parameters, such as level of social distancing, can be highly variable, changing from location to location and from week to week. Other parameters, such as the fraction of infected people who do not develop symptoms, can be difficult to measure. These issues make model fitting extremely challenging. Indeed, connecting the models to data and assessing uncertainty are the most time-consuming and intricate parts of a modelling project.
Can models tell us what groups are the most vulnerable? Where are the weak links in our public health systems? The urgency of these questions explains why we need to develop methods to assess risks before all the evidence is in. Across Canada, many care facilities for elderly people have been inundated by COVID-19, with tragic consequences, while others have escaped unscathed. In Alberta, provincial statistics for numbers of cases have been heavily impacted by two meat-packing plants and, as of early May, these community-scale outbreaks are linked to over one quarter of all reported COVID-19 cases in the province. Similar outbreaks in meat-processing plants have occurred in British Columbia, but many nearby plants have so far avoided infections at this level.
The details of such events cannot be predicted with certainty. Here, we can seek answers from much more complex models that seek to simulate human activity within a computer. Such geographically and socially structured detailed models have previously been developed to predict the spread of pandemic influenza across the USA [5], and the spread of Dengue virus in Southeast Asia [6]. In hindsight, with respect to COVID-19 mathematical models could and should have done much better in this regard. To do better, modellers need to use knowledge and data about the locations, sizes and working conditions within gathering spaces such as factories, prisons and care facilities. This represents a departure from the compartmental paradigm of modelling, where these details are often overlooked in favour of averaging over a whole population.
What about so-called “super-spreader” events? A single 38-year-old patient may have been the first COVID-19 case in Lombardy, a region of Italy at the epicentre of the European outbreak. Italian prosecutors have opened an investigation as to whether delay in his treatment could have triggered a substantial initial surge of infections. Similarly, a large fraction of all cases in South Korea can be linked back to a single church. Studies modelling the impact of super-spreaders on COVID-10 [7], as well as other respiratory diseases such as SARS and influenza, have shown the immense impact of these unpredictable events on disease outcomes. Although we can predict impacts, we are not able to answer the key question for control, which is when and where such events will occur.
To improve the predictive power of mathematical models, we need detailed and rapid data sharing between public health agencies and modelling teams. The data needed depend on the problem to be studied. To understand the rate of spread of the disease among different populations, we need detailed information around the timing of symptom onset, testing, and self-isolation among infected people. To understand relative risks among different age groups, these data must be age-stratified. If we wish to understand the importance of facility outbreaks overlaid on a background of community transmission, we need geographical details of home, work, and events where transmission may be occurring. These data need to be explored by bringing public health officials and modelling teams together, to build understanding of the limitations of field data collection, but also the possibilities that are opened by rapid and detailed data provision.
However, beyond the need for high-quality data, mathematical modelling teams should seek to expand their toolkits to include methods and information streams from outside the classical epidemiology and public health arenas. For example, there have been rapid developments in machine learning and artificial intelligence. These techniques feed on data and generate predictions without making assumptions about mechanisms or hypotheses. As large data sets become available (for instance from much-touted smartphone tracking apps tailored to COVID-19 risk assessment), these methods may become powerful, if blinkered, tools for making short-term predictions. Because the goal of machine learning is simply to predict patterns based on data, approaches that build, for instance, tools to assess credit risk, could also be applied to determine which cities or populations are most at risk.
As a second example, econometricians and government statisticians possess truly impressive amounts of data around business and economic activity, as well as models that can be brought into service alongside epidemiological predictions as we seek to loosen restrictions and reopen our economies. When business sectors return to normal operations, which other sectors are their key suppliers and their essential customers? What if these sectors operate in another province or another country? Which economic sectors and specific businesses have working conditions that pose the greatest risks of driving transmission in the workplace? Which sectors have many employees over 60 years of age, who are therefore at greater risk of serious illness? How well should we expect employees to respect instructions to stay away from work or seek testing if they have mild symptoms? Teams engaged in mathematical modelling of de-escalation should be ready to work with economists and detailed statistical data to understand the complex interplay between economic activity and disease spread.
There is an unprecedented push by scientists worldwide to use mathematical models to understand the workings of COVID-19 spread. The models are being used to make life and death decisions, and Canadians have been at the forefront of the effort, with outstanding groups of researchers working tirelessly. Any successes that the models have in understanding our present predicament owe a debt of gratitude to the painstaking work made by generations of previous mathematical epidemiologists. While a lot has been accomplished in generating new understanding about the initial wave of infection, we still have much work to do, and we can continue to do better.
References
[1] Ferguson, N., et al. Report 9: Impact of non-pharmaceutical interventions (NPIs) to reduce COVID19 mortality and healthcare demand. (2020).
[2] Bernoulli, D. Essai d’une nouvelle analyse de la mortalité causée par la petite vérole. Mém. Math. Phys. Acad. Roy. Sci., Paris, (1766).
[3] Ross. R. The Prevention of Malaria. Murray, London (1911).
[4] Kermack, W.O., McKendrick, A.G. A contribution to the mathematical theory of epidemics. Proc. R. Soc. London, 115:700-721 (1927).
[5] Germann, T.C., Kadau, K., Longini, I.M., Macken, C.A. Mitigation strategies for pandemic influenza in the United States. Proc. Nat. Acad. Sci. USA 103(15):5935-5940 (2006).
[6] Chao, D.L., Halstead, S.B., Halloran, M.E., Longini, I.M. Controlling dengue with vaccines in Thailand. PLoS Negl. Trop. Dis. 6(10):e1876 (2012).
[7] Reich, O., Shalev, G. Kalvari, T. Modeling COVID-19 on a network: super-spreaders, testing and containment. medRxiv https://doi.org/10.1101/2020.04.30.20081828 (2020).
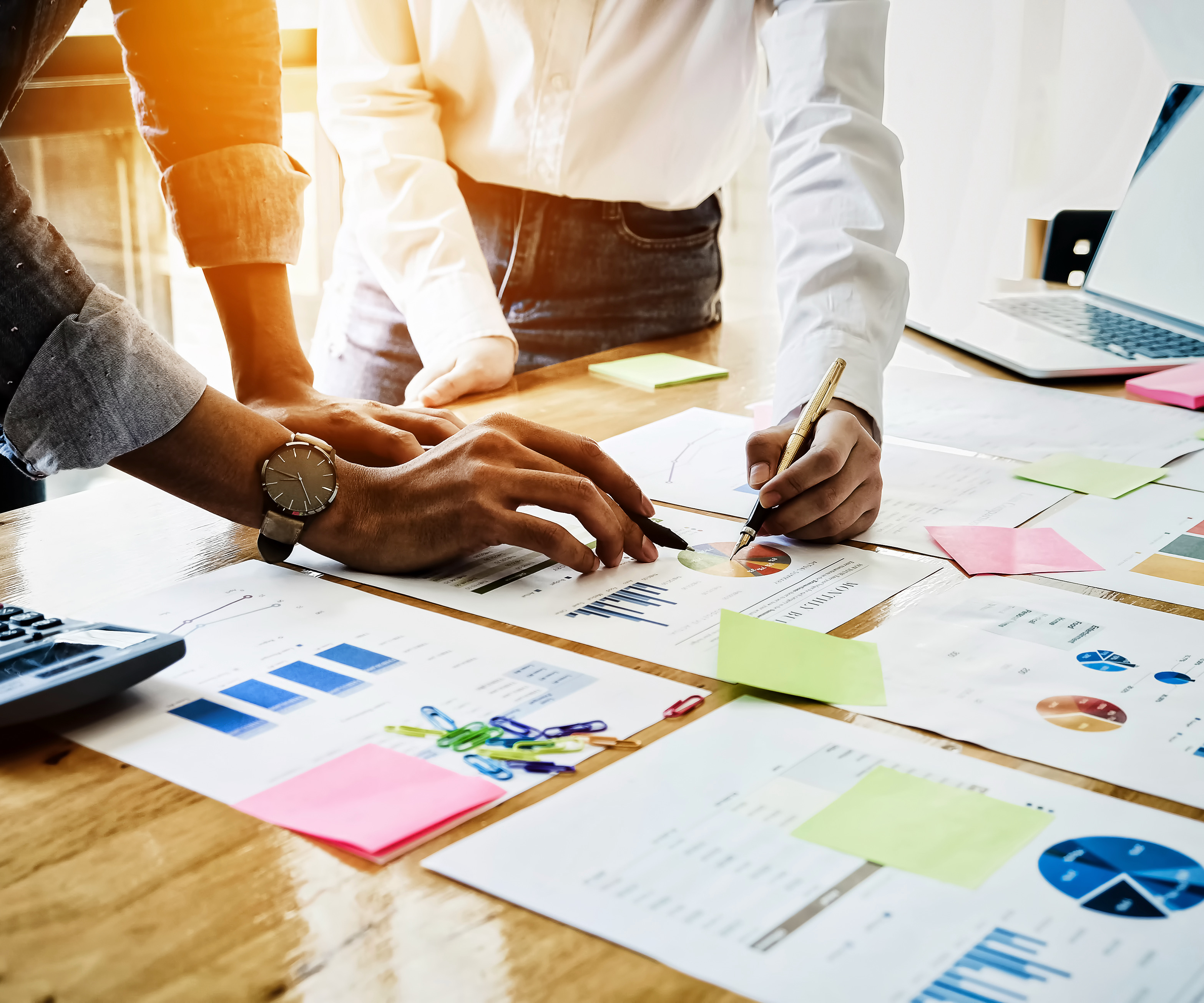