
FLYING BLIND ON COVID-19: TIME TO DO BETTER
Ashleigh Tuite and David Fisman | November 19, 2020
Ashleigh Tuite and David Fisman are epidemiologists and professors at the Dalla Lana School of Public Health at the University of Toronto. Dr. Fisman is also a practising infectious disease physician.
How can one person look at a graph of reported COVID-19 cases and see impending disaster, while another looks at the same graph and feels reassured that everything is just fine? We rely on data to guide and support our decisions. But the COVID-19 pandemic has made it clear: data are not objective. We see data through different lenses, coloured by our existing beliefs and agendas.
At the beginning of the pandemic, we relied on existing public health data collection systems for guidance. These systems are in place to conduct routine disease surveillance, Disease surveillance tells us how many cases of diseases such as whooping cough, flu or syphilis we typically see, and let us know when we are experiencing unusual activity or outbreaks. Their limitations in the face of a pandemic were quickly revealed: manual transcription of information from hand-written collection forms; transfer of information via cutting edge 1980s technologies like fax machines; computer systems that couldn’t talk to each other. We’ve worked to fix many of these problems, demonstrating that we can mobilize solutions quickly when the will exists.
But we haven’t addressed a bigger issue: we are using data and data collection systems that are not designed, and were not intended, to answer the questions we need answered in an urgent manner. Consider a seemly straightforward metric: the number of people currently infected with COVID-19. We receive daily updates on reported case counts; of late, these have been presented by news outlets as “record-breaking”. We tune in to press conferences and are either relieved that case counts appear to be declining or are alarmed that cases are increasing. But these numbers are not a measure of the actual number of infections in our communities.
They depend on how much testing we’re doing and who has access to these tests. If a person doesn’t get tested, whatever the reason, their infection won’t be counted. From a public health systems perspective, changing test criteria as the pandemic progress makes sense, because we need to work within our available capacity and keep our labs functioning. But a constantly changing baseline makes it difficult to interpret the data we have, and to use them to forecast into the near future. The solution to this problem straddles the worlds of research and public health practice. We need data collected quickly, counter to the usual pace of research. But we also need them collected in ways that allow us to answer key questions about true disease burden and risk factors for infections, outside of existing public health systems that are subject to the vicissitudes of testing policy.
Other countries have shown a better way. For example, the United Kingdom which has set up studies to repeatedly test the general population for active and prior COVID-19 infection. Self-collected specimens, which are just starting to come online in Canada, were in use for this purpose in the UK as early as March 2020. Self-collected samples are processed in research labs, to avoid the drain on essential (and limited) public health lab capacity. We can use these sorts of studies to understand how infection patterns are changing over time and better characterize how circumstances like mutigenerational households, poverty, and occupation are contributing to disparities in infection rates. This information can be used to guide our policies: when we see increasing rates in particular neighbourhoods, we can send mobile testing units and determine how else to support these communities before they become hotspots. These types of studies should have been implemented over the summer, but it’s not too late.
We need data on where and how people are getting infected to support policies related to closures and reopening, and provide greater insight into risk factors for disease spread. Again, there are simple study designs that we could use to rapidly collect this information. Test-negative case-control studies, where we survey COVID-19 cases, as well as people who were tested for COVID-19 but whose test comes back negative, can be used to identify risk factors that are more common in cases. This would help us identity the types of activities or exposures that are happening more frequently in cases; from the limited data we have, these risks seem to vary from region to region. Workplaces may be driving transmission in one region, while bars and restaurants are more important in another region. Once we identify these riskier exposures, we can do more thorough investigation to understand what it is about that setting that is enhancing risk. In turn, that information can help us make these settings safer.
With the emergence of vaccines, knowledge about who is getting infected will be all the more important for smart prioritization of regions and groups that are at greatest risk of infection. Having stable, ongoing surveillance systems will remove a lot of the uncertainty that accompanies each change in policy or blip in reported cases.
We are currently trapped in a strange, tautological limbo: politicians say they need to see the data before they act, but the data we are collecting aren’t up to the task of answering fundamental questions. It’s not too late to be smarter, more creative, and more organized in how we surveil this pandemic.
This article initially appeared in the Globe and Mail on November 19, 2020.
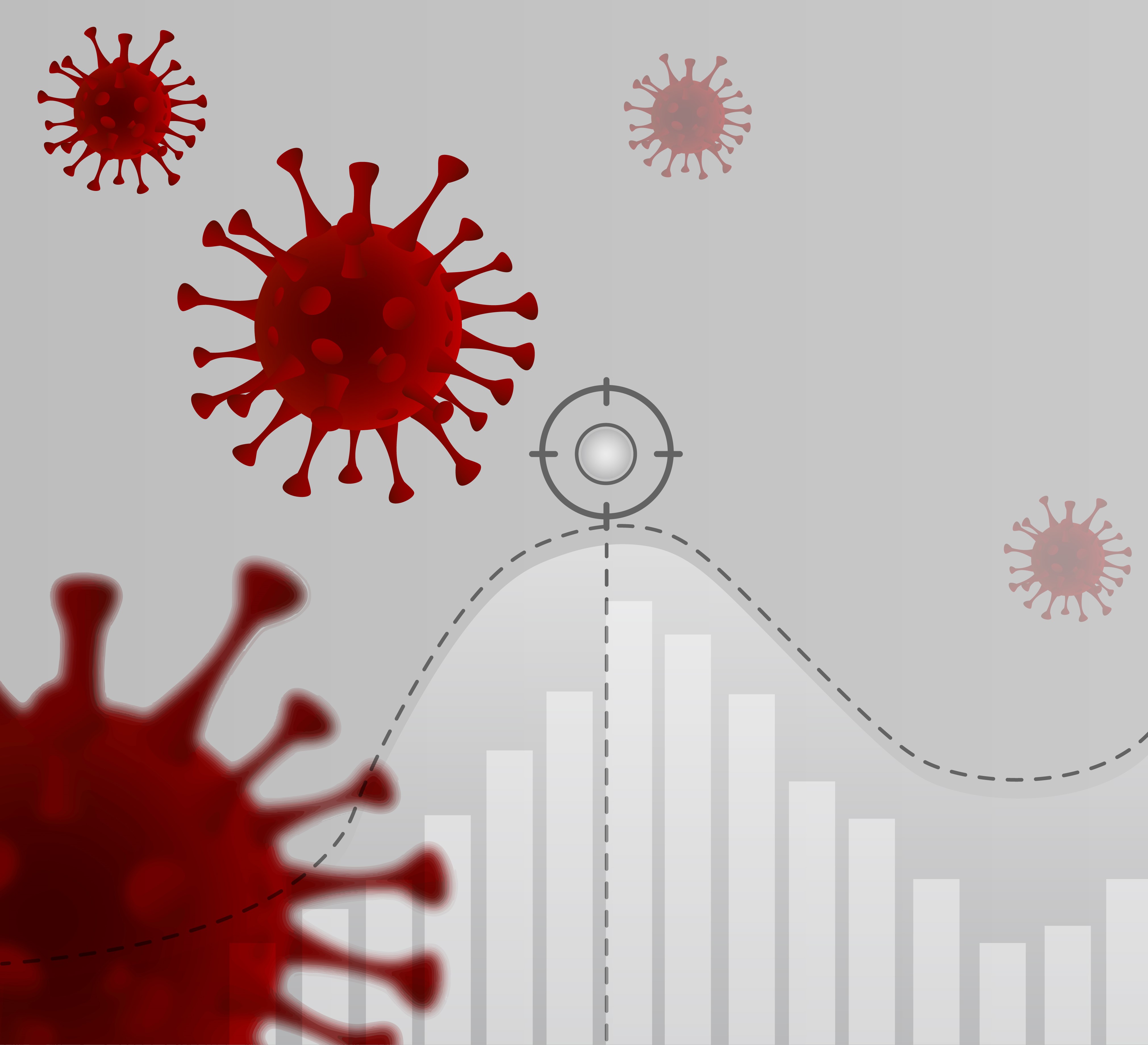